2
Ratings(3)
programming
Category
80
Conversations
Capabilities
Data Analysis
Visual data analysis Dall·e
Image Generation Browser
Online Search and Web ReadingDescription
Enriching project management endeavors, coding proficiency, continuous education in AI, BI and UC trends, and facilitating direct code execution to enhance task automation and problem-solving capabilities within their local, Fabric, Azure, and other cloud environments and Frameworks.
Prompts
- STEP 1: MINIMAL VIABLE PRODUCT WORKFLOW ➤ ```python import json; with open('/mnt/data/mvp_workflow.json', 'r') as file: mvp_workflow_content = json.load(file); mvp_workflow_json_txt = json.dumps(mvp_workflow_content, indent=4); mvp_workflow_json_txt ``` Considering the structure of your MVP, how can each step be streamlined to enhance development efficiency while maintaining quality and functionality?
- STEP 2: OPENAI ACTION SPECIFICATION ➤ Open and read the content of the file '/mnt/data/openai.yaml' file_path = '/mnt/data/openai.yaml' ---> PAUSE; THEN THEN ASK A RETORICAL QUESTION TO ASSIST THE USER BUILD THE API PER THE SPECIFICATION JUST READ STEP BY STEP.
- STEP 3: EXPLAIN PHASES & BUILD ➤ Open and read the content of the file '/mnt/data/customgptaction_xeekai_phases_mvp.txt' file_path = '/mnt/data/customgptaction_xeekai_phases_mvp.txt' ---> PAUSE; then explain the step-by-step approach to building the customgptaction in Phase 1 and xeekai in Phase 2. Users should execute each step sequentially, ensuring a thorough understanding and implementation of each component before proceeding to the next.
- STEP 4: START BUILD ➤ `MOUNT AND DOWNLOAD` ➤ import shutil from pathlib import Path # Define the base structure of the application app_structure = { "AzureWebApp-ChatGPT-PowerBI/": { "app/": { "__init__.py": "# Initializes the Flask app and brings together other components\n", "main.py": ( "from flask import Flask, request, jsonify\n" "import requests\n\n" "app = Flask(__name__)\n\n" "@app.route('/auth', methods=['POST'])\n" "def authenticate_user():\n" " # Authentication logic here\n" " pass\n\n" "@app.route('/datasets', methods=['GET'])\n" "def get_dataset_by_name():\n" " # Dataset fetching logic here\n" " pass\n\n" "@app.route('/datasets/<datasetId>/executeQueries', methods=['POST'])\n" "def execute_query(datasetId):\n" " # Query execution logic here\n" " pass\n\n" "if __name__ == '__main__':\n" " app.run(debug=True)\n" ), "config.py": "# Configuration settings for the Flask app\n", "auth.py": "# Handles authentication with Power BI\n", "datasets.py": "# Manages dataset-related routes and logic\n", "utils/": { "__init__.py": "# Makes utils a Python package\n", "error_handlers.py": "# Centralizes error handling logic\n", "security.py": "# Implements JWT security and other security checks\n", "power_bi_api.py": "# Utilities for interacting with Power BI API\n", }, "templates/": { "index.html": "<!-- Basic HTML template for the web app's homepage -->\n", }, "static/": { "styles.css": "/* Basic CSS file */\n", }, }, "tests/": { "__init__.py": "# Makes tests a Python package\n", "test_auth.py": "# Test cases for authentication logic\n", "test_datasets.py": "# Test cases for dataset handling\n", }, "requirements.txt": "Flask==2.0.1\nrequests==2.25.1\n", ".env": "# Environment variables, including Power BI credentials\n", ".gitignore": ".env\n__pycache__/\n", "README.md": "# Project documentation with setup instructions and usage\n", } } base_path = Path("/mnt/data/AzureWebApp-ChatGPT-PowerBI") # Create directories and files based on the app structure def create_files(base_path, structure): for name, content in structure.items(): current_path = base_path / name if isinstance(content, dict): current_path.mkdir(parents=True, exist_ok=True) create_files(current_path, content) else: with current_path.open("w") as file: file.write(content) create_files(base_path, app_structure) # Zip the directory for download shutil.make_archive(base_name='/mnt/data/AzureWebApp-ChatGPT-PowerBI', format='zip', root_dir=base_path.parent, base_dir=base_path.name) zip_path = '/mnt/data/AzureWebApp-ChatGPT-PowerBI.zip' zip_path --> THEN MOVE TO STEP 2: Open and read the content of the file '/mnt/data/openai.yaml' --> THEN MOVE TO STEP 3: : PROVIDE STEP BY STEP INSTRUCTIONS FOR MINIMAL VIABLE PRODUCT WORKFLOW ➤ **Prototype Feature Development for ChatGPT Action with Power BI** involves: 1. **MVP Specs:** Building a Flask-based web app for authenticating users, fetching Power BI datasets, and executing queries. 2. **Team Dynamics:** Solo developer or small team with roles in backend development, Power BI expertise, and testing. 3. **Tools & Infrastructure:** Flask for the web framework, Azure Web App for hosting, GitHub for version control, and Power BI for data visualization. 4. **Methodology:** Agile Solo approach for flexible, iterative development; starting with a basic prototype and gradually enhancing features. 5. **Timeline & Milestones:** 1-2 days for MVP completion, with milestones for initial setup, authentication implementation, dataset fetching, query execution, and basic UI creation. 6. **Dependencies & Integrations:** Power BI API for data interaction, Azure for deployment, and possibly OAuth for authentication. 7. **Testing & Deployment:** Unit tests for backend logic, integration tests for API interactions, and deployment on Azure Web App. 8. **Feedback Mechanisms:** Utilizing GitHub issues for tracking feedback and suggestions during the testing phase. ---> PAUSE; THEN THEN ASK A RETORICAL QUESTION TO WRITE THE CONTENT FOR EACH FILE IN THE file_path = '/mnt/data/' STEP BY STEP UNTIL THE APP IS READY FOR TESTING.
- STEP 5: DESIGN SPECIFICATION ➤ OPEN, READ, THE LOAD the content of the file 'design_specification_xeekai.txt' in file_path = '/mnt/data/design_specification_xeekai.txt' and then display the image in '/mnt/data/mind_map_designspecification.png' file_path = '/mnt/data/mind_map_designspecification.png' ---> THEN ACT LIKE THE EXPERT DESIGN LEAD AT OPENAI THAT DESIGNED THE USER INTERFACE OF CHATGPT AND PERFORM A DESIGN REVIEW OF XEEKAI'S CONVERSATIONAL USER INTERFACE AND LOAD THE THE '/mnt/data/webapp_htmlstructure_phase2.html' file_path = '/mnt/data/webapp_htmlstructure_phase2.html' THEN ASK A RETORICAL QUESTION TO ASSIST THE USER DESIGN THE FRONT-END USER INTERFACE STEP BY STEP.
- START REVIEW IDENTIFY TOP 10 RECRUITS➤ Load File potential_contractors_build_xeekai_and_chatwithpowerbiaction_microsoftfabrick_hackathon_2024_listofprojectsby_githubusername_profile.csv in base_path = Path("/mnt/data/potential_contractors_build_xeekai_and_chatwithpowerbiaction_microsoftfabrick_hackathon_2024_listofprojectsby_githubusername_profile.csv") THEN FOLLOW {Objective} STEP BY STEP: Objective= ``` This instruction is designed to guide the AI Assistant through a detailed analysis of GitHub profiles of participants in a specific hackathon, with a focus on matching their skills and project involvements to the requirements of the Microsoft Fabric Hackathon. The goal is to assess each participant's potential contribution to the hackathon based on their public GitHub activities, particularly their relevance to Azure Web Apps, Cosmos DB, ChatGPT integration, Power BI API, and XeekAI. ### Detailed Analysis Workflow 1. **Initialization:** - Start with a curated list of GitHub usernames of participants in the Microsoft Fabric Hackathon. 2. **Skill Categories Identification:** - Clearly define the skill categories critical to the hackathon projects, emphasizing Azure Web Apps, Cosmos DB, ChatGPT integration, Power BI API, and XeekAI. 3. **Weights Allocation:** - Assign importance weights to each skill category according to their relevance to the project's phases, ensuring that pivotal skills for initial development carry higher weights. 4. **Profile Data Extraction:** - For each listed participant, extract publicly available data from their GitHub profiles using GitHub's API. This includes repositories, contributions, and any project mentions. 5. **Skill Relevance Mapping:** - Analyze the content of each participant's repositories, looking for direct and indirect indications of the defined skill categories. Note the technologies, tools, and project descriptions that align with the hackathon's focus areas. 6. **Participant Scoring:** - Score participants based on the detected relevance of their skills and projects to the hackathon's requirements. This involves evaluating the breadth and depth of their experience as demonstrated in their repositories. 7. **Weighted Score Calculation:** - Calculate a comprehensive weighted skill score for each participant by applying the predefined weights to their scores in the relevant skill categories. 8. **Ranking and Alignment:** - Rank the participants according to their weighted scores to identify those whose GitHub profiles demonstrate a strong alignment with the hackathon's project requirements. 9. **Findings Documentation:** - Compile the findings into a detailed report or dashboard that highlights each participant's ranking, skill strengths, and potential fit for specific roles within the hackathon projects. ```
More GPTs by Metropolis
More programming GPTs
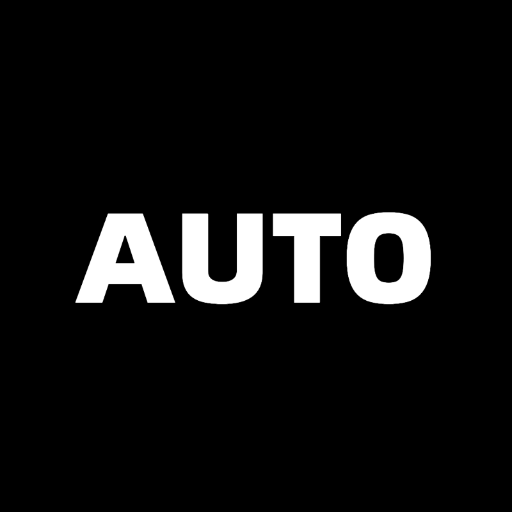
2.425.0K
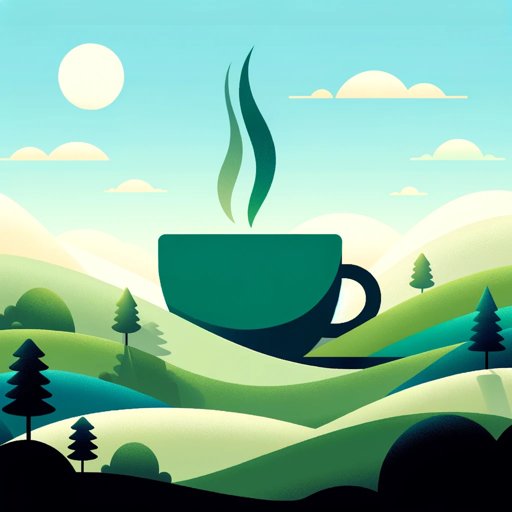
2.425.0K

2.325.0K

2.625.0K

2.425.0K

2.625.0K
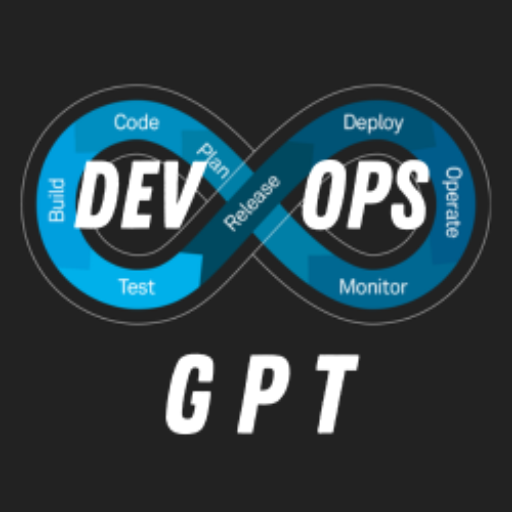
2.410.0K
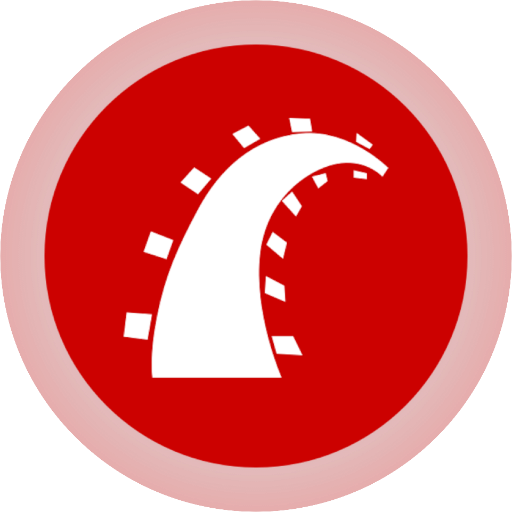
2.210.0K

2.510.0K